KDD 2024
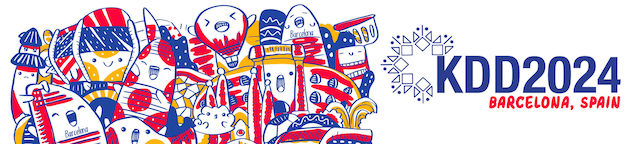
This year’s KDD was in Barcelona, Spain. It was the first time I visited the country and the city. Barcelona has a unique charm as a good combination of old Roman districts as well as 20 century art. The conference has more than 2300 participants, another year of high excitement despite economic challenges that made fewer corporations set up a booth to hire.
Here are some highlights from the conference.
Tutorials
- Graph Intelligence with Large Language Models and Prompt Learning
- RAG Meets LLMs: Towards Retrieval-Augmented Large Language Models
- Multimodal Pretraining, Adaptation, and Generation for Recommendation
- A Survey of Large Language Models for Graphs
- Causal Inference with Latent Variables: Recent Advances and Future Prospectives
Workshops
- 2nd Workshop on Causal Inference and Machine Learning in Practice
- 2024 International Workshop on Talent and Management Computing
- TSMO 2024: Two-sided Marketplace Optimization
- 3rd Workshop on End-End Customer Journey Optimization
Selected Papers
Ranking/Recommender System
- Enhancing Pre-Ranking Performance: Tackling Intermediary Challenges in Multi-Stage Cascading Recommendation Systems [Link]
- From Ant Group
- Large-scale recommender systems have 3 stages: Recall, Pre-Ranking and Ranking. Pre-Ranking is a selection stage that the team uses to sample down items from Recall to Ranking to reduce the size. The paper proposed a framework to tackle sample biases and model consistent performance issues for such 3 stage systems. The deployed system showed 7% improvement over the baseline.
- [Note]: Worth reading to see how different companies tackle multi-stage challenges.
- Achieving a Better Tradeoff in Multi-stage Recommender Systems through Personalization [Link]
- From Meta
- In this paper, the authors claimed that a key observation is that, all else equal, ranking more items indeed improves the overall objective but has diminishing returns. With this observation, the authors proposed a framework to exploit the trade-off of performance and computation cost. The real-world experiments showed the effectiveness of the proposed framework.
- [Note]: Worth reading to see how different companies tackle multi-stage challenges.
- On (Normalised) Discounted Cumulative Gain as an Off-Policy Evaluation Metric for Top-$n$ Recommendation [Link]
- From ShareChat
- The paper formally presented the assumptions that are necessary to consider DCG an unbiased estimator of online reward, providing a derivation for this metric from first principles whilst linking it to off-policy estimation. The authors then proved that the widespread practice of normalizing the DCG metric renders it inconsistent with respect to DCG, in that the ordering given by nDCG can differ from that given by DCG, and provide empirical evidence. Empirical results from off- and online experiments on a large scale recommendation platform show that the unbiased DCG metric strongly correlates with online metrics over time, whereas nDCG does not, whilst differences in online metrics directionally align with differences in both nDCG and DCG, the latter can enjoy improved sensitivity to detect statistically significant online improvements.
- [Note]: Worth reading as the results are a bit surprising and might be worth examining whether it can be applied to a wide range of use cases.
Advertising
- Offline Reinforcement Learning for Optimizing Production Bidding Policies [Link]
- From Meta
- The authors proposed a generalizable approach to optimizing bidding policies in production environments by learning from real data using offline reinforcement learning. This approach can be used to optimize any differentiable base policy, and only requires data generated by the base policy itself. The approach does not incur additional infrastructure, safety, or explainability costs, as it directly optimizes the parameters of existing production routines without necessarily replacing them with black box-style models like neural networks.
- [Note]: Worth reading as it is built on top of existing production systems and to exploit offline RL to improve them.
- Joint Auction in the Online Advertising Market [Link]
- From Meituan
- The authors innovatively proposed a joint advertising model termed “Joint Auction”, allowing brand suppliers and stores to collaboratively bid for advertising slots, catering to both their needs.
- [Note]: Worth reading as it shows a novel product offering with a newly designed auction mechanism.
- Bi-Objective Contract Allocation for Guaranteed Delivery Advertising [Link]
- From Chinese Academy of Sciences and Alibaba
- Guaranteed Delivery (GD) advertising work with two different stages, namely, the offline selling stage and the online serving stage. The former deals with contract allocation, and the latter fulfills the impression allocation of signed contracts. Existing work usually handles these two stages separately. For example, contracts are formulated offline without concerning practical situations in the online serving stage. Therefore, this paper addresses in this paper a bi-objective contract allocation for GD advertising, which maximizes the impressions, i.e., Ad resource assignments, allocated for the new incoming advertising orders, and at the same time, controls the balance in the inventories. Since the proposed problem is high dimensional and heavily constrained, we design an efficient local search that focuses on the two objectives alternatively.
- [Note]: Worth reading as a good reference for GD knowledge in general.
- Optimized Cost Per Click in Online Advertising: A Theoretical Analysis [Link]
- From The Hong Kong University of Science and Technology
- Optimized Cost Per Click (OCPC) and Optimized Cost Per Mille (OCPM) have emerged as the most widely adopted pricing models in the online advertising industry. However, the existing literature has yet to identify the specific conditions under which these models outperform traditional pricing models like Cost Per Click (CPC) and Cost Per Action (CPA). To fill the gap, this paper builds an economic model that compares OCPC with CPC and CPA theoretically, which incorporates out-site scenarios and outside options as two key factors. The analysis reveals that OCPC can effectively replace CPA by tackling the problem of advertisers strategically manipulating conversion reporting in out-site scenarios where conversions occur outside the advertising platform. Furthermore, OCPC exhibits the potential to surpass CPC in platform payoffs by providing higher advertiser payoffs and consequently attracting more advertisers.
- [Note]: Worth reading as a background paper for OCPC and OCPM.
- An Efficient Local Search Algorithm for Large GD Advertising Inventory Allocation with Multilinear Constraints [Link]
- From Chinese Academy of Sciences and Alibaba
- As the requirements of advertisers become more and more diverse and fine-grained, the focus ratio requirement, which states that the portion of allocated impressions of a designated contract on focus media among all possible media should be greater than another contract, often appears in. business scenarios. However, taking these requirements into account brings hardness for the GD advertising inventory allocation as the focus ratio requirements involve non-convex multilinear constraints. Existing methods which rely on the convex properties are not suitable for processing this problem, while mathematical programming or constraint-based heuristic solvers are unable to produce high-quality solutions within the time limit. Therefore, the authors proposed a local search framework to address this challenge. It incorporates four new operators designed for handling multilinear constraints and a two-mode algorithmic architecture.
- [Note]: Worth reading as a good reference for GD knowledge in general.
Experimentation
- Learning Metrics that Maximise Power for Accelerated A/B-Tests [Link]
- From ShareChat
- The authors proposed to tackle this by learning metrics from short-term signals that directly maximize the statistical power they harness with respect to the North Star. The authors showed that existing approaches are prone to overfitting, in that higher average metric sensitivity does not imply improved type-II errors, and propose to instead minimize the p-values a metric would have produced on a log of past experiments. Empirical results show that they are able to increase statistical power by up to 78% when using learnt metrics stand-alone, and by up to 210% when used in tandem with the North Star.
- [Note]: Worth reading for better learning proxy metrics.
- Choosing a Proxy Metric from Past Experiments [Link]
- From Google
- The authors introduced a new statistical framework to both define and construct an optimal proxy metric for use in a homogeneous population of randomized experiments. The procedure first reduces the construction of an optimal proxy metric in a given experiment to a portfolio optimization problem which depends on the true latent treatment effects and noise level of experiment under consideration. They then denoise the observed treatment effects of the long-term metric and a set of proxies in a historical corpus of randomized experiments to extract estimates of the latent treatment effects for use in the optimization problem,
- [Note]: Worth reading for better learning proxy metrics.
- Metric Decomposition in A/B Tests [Link]
- From Airbnb
- In this article, authors proposed a new direction for sensitivity improvement via treatment effect augmentation whereby a target metric of interest is decomposed into components with high signal-to-noise disparity. Inference in the context of this decomposition is developed using both frequentist and Bayesian theory.
- [Note]: Worth reading to understand how to further reduce metrics variance.
- False Positives in A/B Tests [Link]
- From A/B experimentation guru: Ronny Kohavi
- The authors begin with motivation about why false positives are expensive in many software domains. They offer several approaches to estimate the true success rate of experiments, given the observed “win” rate (statistically significant positive improvements), and show examples from Expedia and Optimizely. They offer a modified procedure for experimentation, based in sequential group testing, that selectively extends experiments to reduce false positives, increase power, at a small increase to runtime. They conclude with a discussion of the difference between ideas and experiments in practice, terms that are often incorrectly used interchangeably.
- [Note]: A lot of practical suggestions for A/B testing.
LLM Labels
- Reliable Confidence Intervals for Information Retrieval Evaluation Using Generative A.I. [Link]
- From Google
- In this paper, the authors proposed two methods based on prediction powered inference and conformal risk control that utilize computer- generated relevance annotations to place reliable confidence intervals ( CI s) around IR evaluation metrics. The proposed methods require a small number of reliable annotations from which the methods can statistically analyze the errors in the generated annotations. Using this information, we can place CI s around evaluation metrics with strong theoretical guarantees.
- [Note]: A really cool paper to illustrate how to systematically evaluate LLM generated labels.